Safety arguments and confidence quantification
The aim is to help build a safety case using formalized models. The work is based on Bayesian networks and belief functions (Dempster-Shafer theory).
Building a safety case is one way of preparing critical systems for certification.This mainly involves justifying how each hazard has been dealt with and reduced to an acceptable level.Unfortunately, in the case of systems with many uncertainties and not covered by standards (such as autonomous vehicles), there is currently no systematic method for building such safety cases and demonstrating the underlying level of confidence.Our work aims to contribute to the definition of such a method by starting from risk analysis techniques, then using formalized models to build the safety case and automatically quantify the level of confidence in this case.
Q.-A. Do Hoang's thesis linked the HAZOP-UML[1] hazard analysis technique to safety argument models (in Goal Structuring Notation, GSN). On the basis of these models, an initial work using Bayesian networks enabled us to associate a quantitative assessment of confidence with the elements of the argument, and to propagate this to the final decision[2]. This work was followed by R. Wang's thesis[3],[4], which focused on the use of belief functions (Dempster-Shafer (DS) theory), to evaluate and propagate trust in a GSN.Two important challenges were addressed:
- How can trust be formally defined? Uncertainties exist in the argument designed to demonstrate system safety. Confidence can be achieved by measuring these uncertainties. Assessing uncertainties in an argument put forward by experts is often subjective, but we have proposed a quantitative model based on the 3 quantities disbelief, uncertainty and belief from DS theory.
- How to aggregate and propagate confidence levels? Aggregation rules are essential for propagating confidence in an argument. For example, several premises referring to the same statement may be complementary or redundant. We have proposed new mathematical rules for aggregating confidence, based on the tools of belief functions.
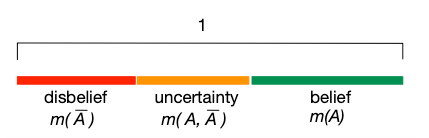
[1] Guiochet J., Hazard analysis of human–robot interactions with HAZOP–UML. Safety Science, vol. 84, pp. 225-237, 2016. Elsevier
[2] Guiochet J., Do Hoang Q.-A., Kaâniche M., A Model for Safety Case Confidence Assessment. 34th Int. Conference on Computer Safety, Reliability and Security (SAFECOMP-2015), Delft, The Netherlands, 2015
[3] Wang R., Guiochet J., Motet G., W. Schön, Modelling Confidence in Railway Safety Case. Safety Science, 110 part B, pp. 286-299, 2018.
[4] Wang R., Guiochet J., Motet G., Schon W., Safety Case Confidence Propagation Based on Dempster-Shafer theory. Int. Journal of Approximate Reasoning, vol. 107, pp. 46-64, 2019. Elsevier